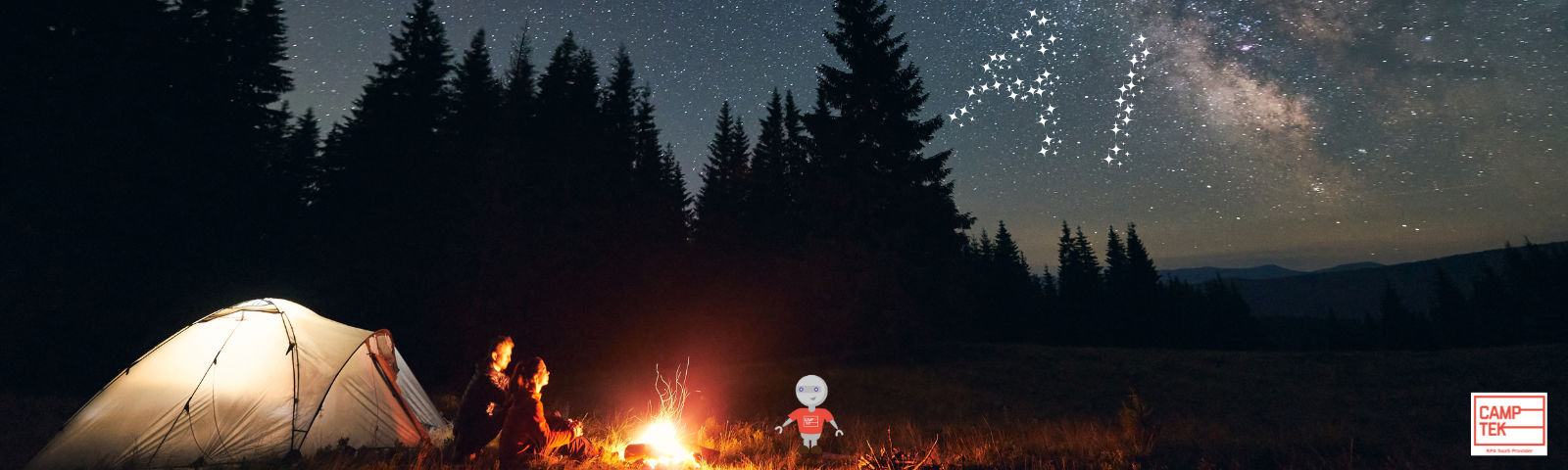
Article for reference: Why humans will never understand AI – BBC Future
There are generally two outlooks on AI. One is of hope and excitement for the future and the endless possibilities. The other is the fear of a doomsday scenario where we serve an AI overlord. The tone of Beers’ writing leads me to believe he is in the doomsday camp. For example, he quotes Hardesty, who wrote an explanation of neural networks in 2017. He touched on most of the points Beers does, but the reader is left with a more positive outlook (“Explained: Neural Networks”).
The only piece of deep learning that isn’t fully understood is the invisible or hidden layers. He touches on this with corroboration, but the reader (well me anyways) is led to believe that everything in between the input and output layers is mystical and unknown. That isn’t the case. The number of nodes and layers are defined by the network’s creator. These parameters are determined by the complexity of the problem being solved or application use case. Ahmed Gad published a piece on one of my favorite sites, Towards Data Science, that illustrates the methodology in making these choices (Gad). How data makes it through the layers and nodes is also well known, albeit PHD level math and makes my head hurt just looking at it.
The real unknown is why the data takes the path that it does becoming radically transformed from input to output. There are several theories of the why, which are well above my level of understanding, but haven’t been accepted. I have a theory that someone knows exactly how it works, but like Galileo, has been deemed preposterous until eventually proven true.
Neural networks have been commonplace in modern life for quite some time. Google searches have relied on deep learning since 2015. Siri and Alexa are widely used neural network applications. There have been issues raised in regard to those, but mainly along the lines of privacy. All of this begs the question, “Why all the hype now?” Tomaso Poggio theorized that “ideas in science are a bit like epidemics of viruses”. The hype and fear of an idea comes and goes in cycles. So, is the excitement over ChatGPT just another cycle? Has the threat of cheating or writing papers and reports with little effort fueled this fire? The fear of AI robots replacing human jobs has existed for decades.
I see the biggest threat being an accentuation of an existing issue with humans. Misinformation and propaganda campaigns are very effective tools. A portion of the populace will give merit to something on face value from a social network. Now there are numerous tools that allow threat actors to generate misinformation presented by credible sources via audio and video. Pieces that will be very difficult for those same people to discern fact from fiction. That has already led to new business opportunities in detecting the presence of a deep fake.
…or we could end up as batteries for the robots in vats of gelatin. Time will tell.
Response by: Dennis Stone, Senior Dev Ops Engineer
“Explained: Neural Networks.” MIT News | Massachusetts Institute of Technology, 14 Apr. 2017, news.mit.edu/2017/explained-neural-networks-deep-learning-0414.
Gad, Ahmed. “Beginners Ask ‘How Many Hidden Layers/Neurons to Use in Artificial Neural Networks?’” Medium, 27 June 2018, towardsdatascience.com/beginners-ask-how-many-hidden-layers-neurons-to-use-in-artificial-neural-networks-51466afa0d3e